REBECCA project wins the Best Paper Award at AIAI 2022
On 19 June, Ms Niki Kiriakidou, Harokopio University of Athens, represented the REBECCA project at the 18th International Conference on Artificial Intelligence Applications and Innovations (AIAI 2022) with a paper titled An improved neural network model for treatment effect estimation. The paper has received the Best Paper Award, assigned by the General and Program Co-Chairs of the conference.
This year’s edition of AIAI was held as a hybrid event on 17-20 June in Crete, Greece. The event was hosted by the Democritus University of Thrace and the University of Piraeus, in cooperation with Springer, IFIP, and Easy Conferences. Since 2005, when the event first took place, it has grown to be the meeting point for leading scientists from Europe and beyond.
The paper An improved neural network model for treatment effect estimation, authored by Ms Niki Kiriakidou and Dr Christos Diou, looks at the proposed neural network model, which achieved better estimation of causal effects than the state-of-the-art models, i.e. Dragonnet.
Randomised Controlled Trials (RCTs) are the gold standard for calculating the true causal effect of an intervention. RCTs is a study design that randomly assigns participants into an experimental group or a control group. While this study design has proven to be highly valuable and necessary, it has certain limitations. RCTs are expensive, time-consuming, and have ethical restrictions.
Observational data present a tempting shortcut to RCTs when it comes to estimating causal effects. In her presentation, Ms Kiriakidou underlined the value of using the plethora of observational data in estimating the effect of an intervention. Observational data refers to information gathered without the subject of the research having to be explicitly involved in recording what they are doing. In this particular case, in order to evaluate the proposed and state-of-the-art models, researchers used the semi-synthetic IHDP dataset constructed from the Infant Health and Development Programme.
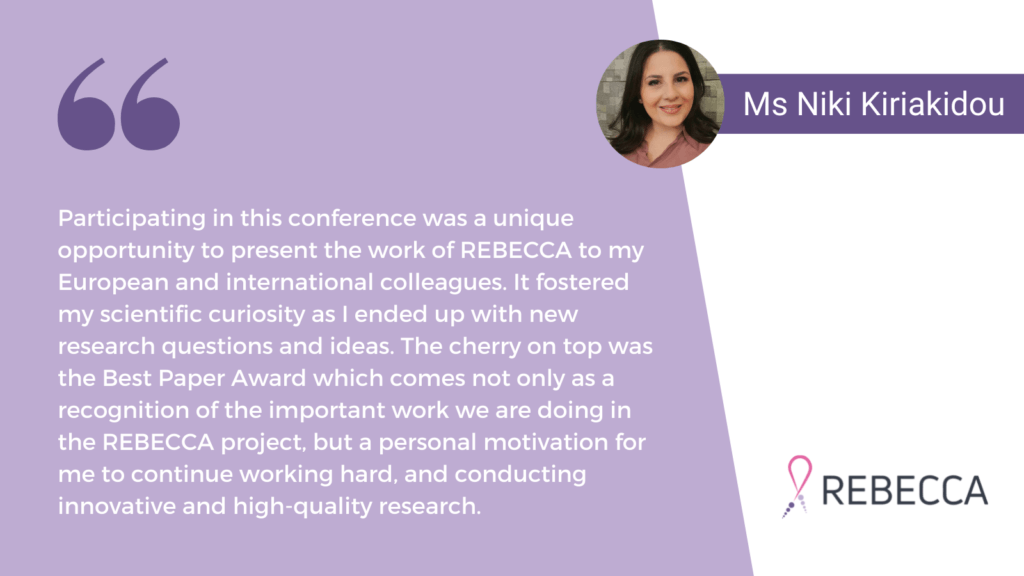
The paper presented by Ms Kiriakidou features a new neural network model, Modified Dragonnet, which provides more accurate predictions of treatment effects than the state-of-the-art models, not only for the general population but also for individuals. A neural network is a series of algorithms that mimic the operations of the human brain to recognise relationships between vast amounts of data. As such, they tend to resemble the connections of neurons and synapses found in the brain.
Modified Dragonnet, will be used in conjunction with other state-of-the-art models in the REBECCA clinical studies which will be conducted in Norway, Sweden, and Spain. These studies will look at the impact of breast cancer treatment on patients’ functional and emotional status, in particular, the prevalence of fatigue, osteopenia and peripheral neuropathy. The studies will involve over 650 individuals and will help shape future guidelines and practices for post-cancer treatment.